Dynamic hierarchical bayesian inference underpins human social learning
Seminar
- Date: Jun 2, 2016
- Time: 01:00 PM - 02:00 PM (Local Time Germany)
- Speaker: Dr Andreea Diaconescu
- Translational Neuromodeling Unit (TNU) University of Zürich/ETH Zürich
- Location: Max Planck Institute of Psychiatry
- Room: Lecture Hall
- Host: Leonhard Schilbach
- Contact: leonhard_schilbach@psych.mpg.de
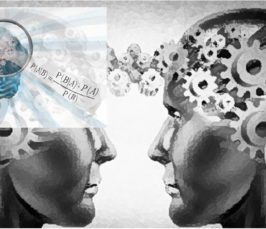
Information about the state of the world reaches the brain through the senses. In contrast to the traditional view postulating that sensory information propagates through the brain in a feed;forward manner, it has recently been suggested that perception is as an active process with the brain constantly generating hypotheses about the causes of its sensory inputs in order to anticipate future events.
This notion that the brain continuously updates a generative model of the causes of its sensory inputs has been formalized under the “Bayesian brain hypothesis”. This proposal is particularly critical for social learning when predictions about the causes of social stimuli become building blocks for more complex representations of other persons, including intentionality. In this talk, I will present evidence from three neuroimaging studies employing either fMRI or EEG in the context of social learning, that the brain utilizes Bayesian inference machinery, in particular hierarchically organised precision;weighted prediction errors, to generate a model of another person and his/her intentions.